So far, almost 1 million people have died as a result of contracting COVID-19. And over 30 million have contracted the disease already. Across the world, countries are recording a renewed spike in cases. The solution to controlling the pandemic, as suggested by healthcare experts around the world, is to reduce R0. This can be done by ramping up testing so those who catch the infection can be isolated and treated effectively. But doing this comes with challenges.
For one, test kits are expensive. And along with tests come the added cost of infrastructure and specialised equipment. This is especially true in rural and remote areas around the world. Even if this is overcome, the test that is considered gold standard–Real-time polymerase chain reaction (RT-PCR)–has a long lead time. Added to the mix is that Covid-19 symptoms–cough, fever, fatigue–are not unique and can be brought on by various illnesses.
And until there is a vaccine or a cure for this disease, there will be a continuous need for wider testing. This makes the effective utilisation of resources extremely important.
This brings with it a need to create a screening layer, which can potentially identify those who have a high probability of testing negative for Covid-19. Our effort, Cough against COVID, could help. We demonstrate that solicited cough sounds have a COVID signature, including asymptomatic patients. At our current performance, when our model is used as a screening layer, before RT-PCR, it will increase testing capacity by 1.43X without increasing the number of lab-based tests.
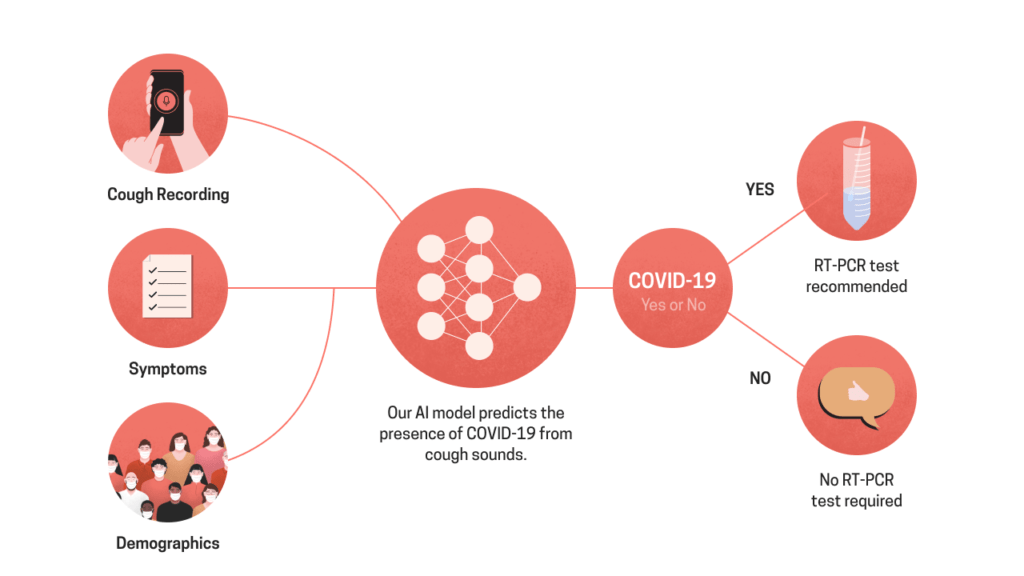
How does it work?
Physicians have used sound as an identifier in respiratory illnesses. But these sounds, especially cough, are not easily quantifiable for a layman. When we began our research, our contention was that AI may be able to identify this sound. We collected over 3,500 solicited-cough sounds from COVID centres across India. These included both positive and negatives, confirmed by an RT-PCR test. While the data collection is ongoing, we used a curated set of about 1000 individuals to present the scientific analysis.
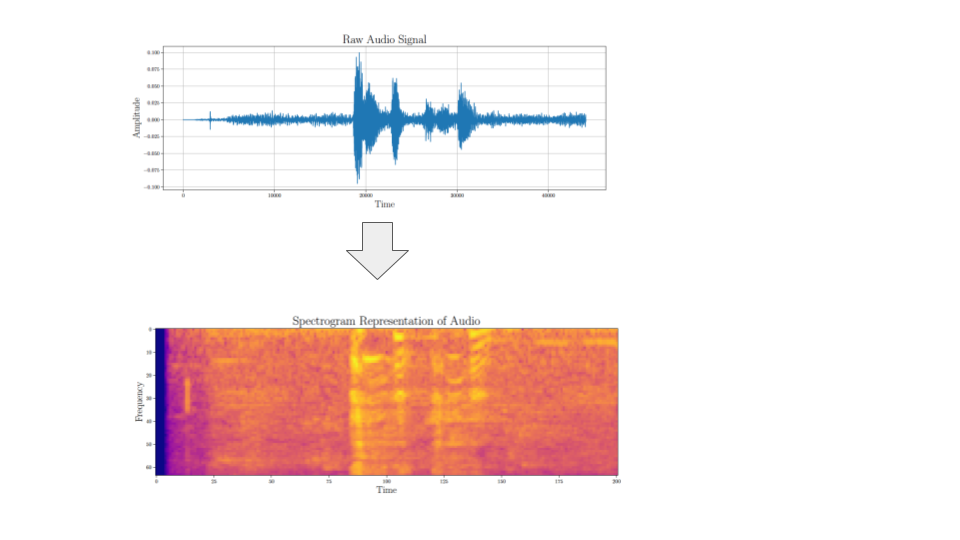
The first step is to convert cough sounds into a model-ingestible representation. It is well known that spectrogram-based representations of sound work well in practice, especially with convolutional neural networks (CNNs). CNNs are extremely versatile, multi-step function approximators known to work exceedingly well on images.
We developed an end-to-end CNN-based framework that ingests audio samples as spectrograms and directly predicts a binary classification label indicating the probability of the presence of COVID-19.
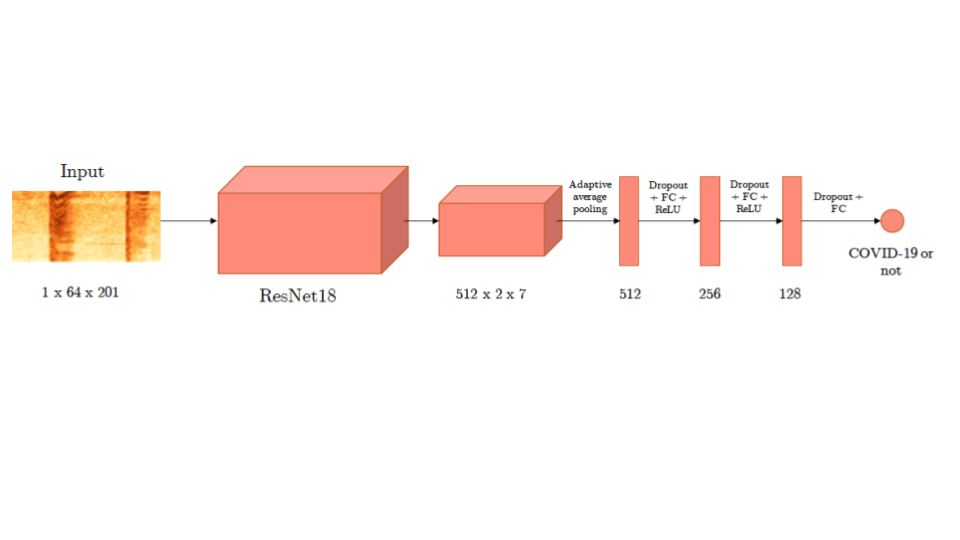
Our model architecture was first pre-trained on open-source cough datasets to simply predict the presence of a cough (cough detection). Next, we trained our model on the primary task of COVID-19 detection. During training, we use standard augmentation techniques and smoothened labels to make our model robust to input noise and label noise arising due to imperfect RT-PCR test results.
Our model, with statistical significance, demonstrates that solicited-cough sounds have a detectable COVID-19 signature, and this holds true even for asymptomatic individuals.
This could hence be a model that can be used to reliably detect COVID-19 negative individuals while we refer the positives for a confirmatory RT-PCR test. In this way, we increase the testing capacity by 43% (a 1.43x lift) when we assume a disease prevalence of 5%.
Possible ways to deploy
It can work on a basic feature phone and doesn’t require skilled personnel. It is inherently designed to scale naturally, without additional work.
Another possible way our model can be deployed is via apps on a smartphone of healthcare workers, helplines or via voice notes on instant messaging apps. Our data collection, however, is ongoing, and subsequent models will be trained on individuals beyond the subset in this study. We will also explore fast and computationally efficient inference, to enable COVID-19 testing on smartphones. This will enable large sections of the population to self-screen, support proactive testing and allow continuous monitoring.