In December of last year, we reached Bharuch in Gujarat. A five-hour journey from Mumbai to Vadodara via a flight and then a drive. Masked and socially distant. This was our third visit to access how healthcare workers weigh babies. It was not the way we wanted to conduct our usability testing.
For the past three years, Wadhwani Institute for Artificial Intelligence has been trying to solve a problem: to create a solution where one can detect low-weight newborns by taking a short video on a smartphone. This would enable a system where frontline workers could prescribe home-based care to these newborns or refer them for medical intervention. We detailed the problems that frontline workers face in an earlier post.
With this problem set in mind, we spent our first two visits (pre-Covid) along with our usability partner Navana Tech, recognising the challenges and understanding the limitations of our product usability. The third, with operational support from SEWA Rural, was to use the learnings from the first two times on the field, create a prototype and test it with frontline workers. There was some trepidation that frontline workers would find the process and the interface cumbersome and would reject it.
But our fears were unfounded. Not only were the prototypes widely accepted but many of our existing data collecting frontline workers couldn’t wait to use it in their application.
What we did
We first went to Bharuch in 2019. The visit was just to understand the landscape. On this trip, all we wanted to do was immerse ourselves in the environment and pick up nuances. Our second visit to Udaipur, Rajasthan, was at the beginning of 2020. This time, we picked up details that would help us develop our app. For example, we realised that we could not rely on the lights available in the homes. The app would need to account for low light. Our solution needs a baby to be laid on an even, flat surface. We learnt that the ground the baby is placed on may not be level. Another was that mothers may not want to leave their baby uncovered because of the extreme temperatures. Some of these challenges technology could solve, some needed human intervention.
Our third visit was set for mid-2020 when the pandemic struck. We had planned to conduct extensive research and surveys to understand not just the way the app was used, but also types of phones it might be installed on, features such as camera quality and the comfort level frontline workers had with their phones.
We did conduct ad-hoc surveys by using surrogates for frontline workers but it was not the same. We had lost time. So, when we travelled in December, we were tentative.
Across three days we had planned to meet 18 ASHAs. We had taken two prototypes. The first prototype relied on using the gyroscope found in slightly higher-end phones to provide frontline workers with real-time feedback. The second prototype did not use the gyroscope and relied on providing fixed time-based nudges to capture the video successfully. While we were aware that most phones used by frontline workers today do not support gyroscopes, we wanted to be forward compatible and build for old, basic phones as well as new emerging low-cost smartphones.
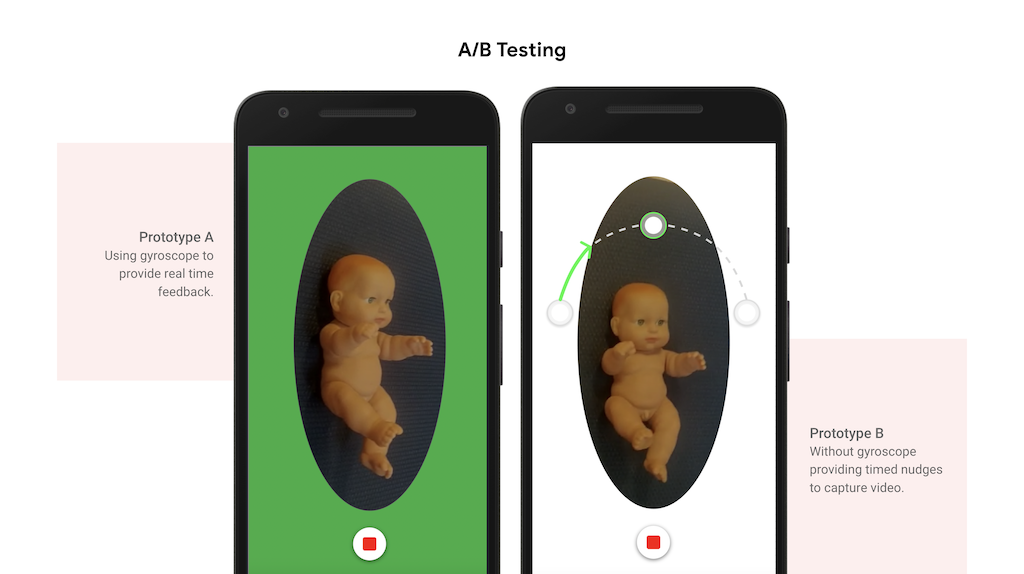
We hoped that the ASHAs would take to one of these prototypes and we would use that as our base to build our solution. Each day had a specific goal. On day one, we showed six ASHAs our prototype. Three used prototype A and the rest used B. On day two and three, 12 ASHAs used both prototypes and were asked if they preferred one over the other.
We handed these phones to ASHAs and asked them to use these apps on dummy subjects.
And that’s when we met Laxmiben. She was reluctant to participate in the study, calling it a waste of time. It took a lot of coaxing and convincing to give it a test drive. She took to it almost immediately. In fact, by the end of it, she asked when our app would be ready to download from the store and if she could use the app to collect data for us.
All of this when the app doesn’t even give results yet – it just nudges the ASHAs on how to make a video.
What we captured
We asked ASHAs to take videos by drawing an ‘arc’ with their smartphones around the baby. This required them to bend and get close to the babies. While they conducted these experiments, we recorded the sessions. During this, we also captured metadata, for example, how much the frontline workers bent, how much of the baby got captured, and the way they interacted with the app. We also captured them performing the task and documented what aspects frontline workers liked and what they didn’t.
Each video taken by ASHAs was then scored by the research, the design and the product teams. The scores indicated how well they had captured the baby – if side angles were captured, if the baby was cropped and if the arc was smooth.
Now, comes the exciting part. Our research, product and engineering teams will take what we learnt on and work in conjunction to create a solution powered by AI. This could potentially help millions of babies across the world.
Our colleagues have been documenting how they approach building the model in a series of posts. You can read them here and here.