In my earlier article about why tech solutions should focus on low-income users, I argued that although not everyone may need training manuals to use tech-based solutions, they can go a long way towards supporting rural users such as farmers who are not entirely familiar with technology and its uses in agriculture.
Let me recount our team’s efforts on this front so far. In addition to creating learning materials to use on the field, we also conducted a survey (referred to as an ‘adoption study’ internally) to understand what was needed to make it successful.
What have we tried already?
Remote training using slide decks: The COVID-19 pandemic has forced us to learn to communicate from a distance, but Zoom calls have helped us retain some emotional and professional connections. We carried out a training session for key personnel from partner organisations, including team and PU managers, field facilitators, and lead/ cascade farmers. This was an hour-long Zoom call, in which an overview of the problem, the solution, and its impact was explored.
Human-centred design approach: I reviewed the deck and listened to the recordings of the calls and realised that it was not the best way to train those users. It might have worked as an introduction, but they needed more in-person training. Users confirmed this observation during another adoption study.
In-person demonstration: A session to demonstrate the app’s functionality was also organised, in which farmers and partners learned , step-by-step, how to use the app on the field—installing traps, taking photos on the app, understanding alerts, and using advisories.
Human-centred design approach: When I interviewed the users later , this method was remembered as a success; everybody was happy and had gained enough confidence to try using the app themselves!
Printed training material (work in progress): Training material has been prepared in the past but not tested in the field. We decided to review it from an HCD perspective and found some opportunities to improve the material.
Human-centred design approach: As per our design review, we had a few clear needs in terms of training material:
- User–specific material which will be tailored to the two different types of learners in this context—farmers and field facilitators
- Printed material to make it easy for farmers to refer to it while using the app.
- Training manual kit for the solution, not just the app. Our solution is app-based but it is not limited to the app. There will be many different outcomes and effects based on the decisions users make, and we need to address these in the training manuals.
What could be improved
One–time training: Our team had partial success with training through the on-field demo, but it happened only once. Farmers and field facilitators kept sharing that this format allowed space for learning and asking questions that wouldn’t be possible otherwise.
The same type of training for everyone: Remote training did not have a larger impact because though the participants consisted of different types of users, the presentation was created keeping partner organisations in mind. We must recognise the varied needs and interests that exist within our audience, in order to act inclusively. This seems like an easy problem to solve, but it is not. Keeping everyone engaged and focused on the same objective is a tough task. We are looking to implement a more participative method of training.
Training manuals that are only accessible on phones: While a part of the training audience was technologically savvy and had already been using apps in their day-to-day lives, farmers rarely had the time to explore their apps, mostly using non-farming related ones when they could. They used their phones to watch videos and get weather updates. This little bit of familiarity allowed them to take some interest in the presentation, but it was not enough to keep them engaged for long..
It made us realise that we need to leave something tangible with them post-training which they can refer to or learn from. It could be a tool or some reading material, something they can touch and hold. One objective of such a tool would be to kickstart deeper engagement and more regular feedback, which will help us build trust with those who may benefit from our work.
No room for dialogue: The kind of training we conducted remotely did not leave room for dialogue, which is why the on-field demo was so much more successful.
It is important for us to provide consistent support to low-income users so that they can ask questions, give us feedback, and participate in designing newer and better features.
Reflection: While we have identified specific methods which worked or didn’t work, we also need to adopt an approach which is driven by reflecting on gaps in our systems and processes, asking questions, and understanding users.
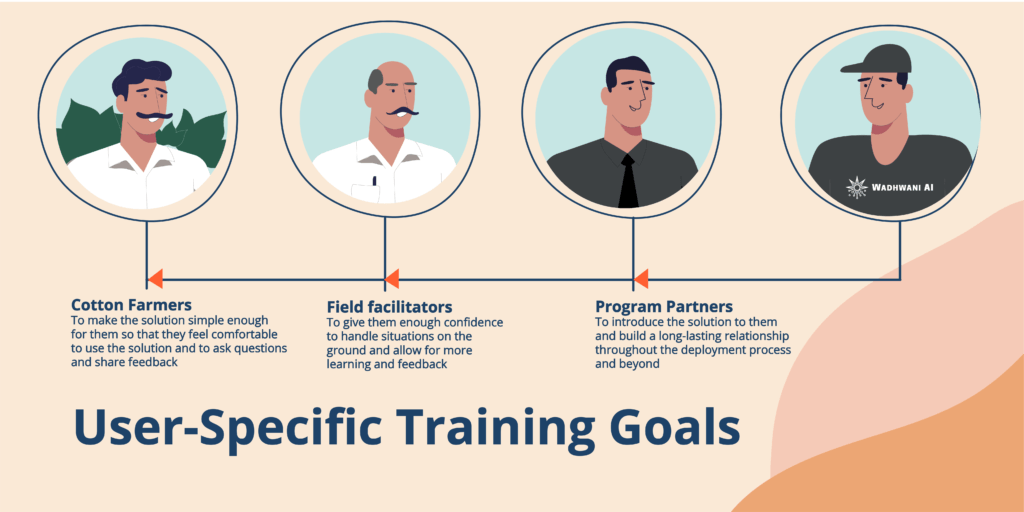
How are we moving forward?
Training for different user groups: We are now preparing training material that can engage different types of users, such as program partners, field facilitators, and farmers. We are also working on equipping our trainers better so that they can teach users more engagingly and effectively.
Clear training goals for user groups:
- Partners: Our goal is to introduce the solution to them and build long-lasting relationships throughout the deployment and beyond.
- Field facilitators: Our goal is to give them enough confidence to handle situations on the ground and allow for more learning and sharing of feedback
- Farmers: Our goal is to make the solution simple enough that they are comfortable implementing it in their own way, and to develop a relationship of trust, in which they feel welcome to ask questions and share their feedback.
Regular and frequent training: There is a need to ensure that enough training material is created so that there is space for regular training sessions, based on various user needs and the feedback shared by our users. We are working on this.
YouTube knowledge centre: We learned from an adoption study that farmers who use apps on their phones consume a lot of content on YouTube when they have some free time. Some users shared that they love to watch agriculture experts talking about new seeds, new pesticides, and new practices, which farmers are adopting across the country. Some of them also shared that they have subscribed to these YouTube channels and follow them regularly.
Is this surprising? Not really. Visual content is more engaging and can aid in long-lasting learning. We found many organisations which are engaging farmers on YouTube. We are currently building a knowledge centre in the form of a YouTube channel and will find ways to engage cotton farmers.
On-field use cases and recommendations: Our user manuals cover information about the solution, monitoring guidelines, etc., but we learned that our farmers have been experimenting with the app and their traps because they are curious and want to test the technology before they trust it. It sounds fair, doesn’t it? But it has also added an extra burden on the field facilitators to answer unexpected questions they were not trained for. We have included such field experiences and user scenarios along with recommendations on how to navigate them. This is primarily for the benefit of the field facilitators, so they can gain enough confidence in order to answer difficult questions. As an organisation, we will keep evolving as we learn more.
Farmer’s stories: The farmers’ trust in the solution is paramount. Why would they trust something they have never seen or tried before? We noticed how farmers share stories with each other about bad experiences due to pest attacks. They also talk about their visits to agricultural fairs and doctors, who themselves are farmers, to learn about new practices. This inspired us to add a section titled “Farmers’ stories” in the farmers’ version of the training manual.
As we continue to reflect on our work, designing more inclusive processes that will allow our users to reflect with us will only make our vision clearer. Learning about specific challenges farmers face, not just in using app-based solutions, but even in accessing them, has helped us to better understand how to address their needs.