At Wadhwani Institute for Artificial Intelligence, we work on finding elegant solutions to difficult problems. One such problem is the identification of low birth weight babies. We detailed the role health workers play in a post last year. And in an earlier post, we identified the role AI can play and an overview of the challenges that lie in front of us.
Today, we want to wade deeper into the pool of how AI can take the next step. In this post, we want to identify how we collect 2D data, set up the data pipeline, process the data to make it usable and the challenges that we encounter in the process.
Data is one of the most important building blocks of any AI model. Collecting good quality data and annotations is very important for any data-based modelling. The saying goes “Garbage in, Garbage out” so it’s important that the data and labels convey information that is relevant and useful for the model, otherwise the predictions are as good as garbage. Hence, it is wise to spend a fair amount of time upfront figuring out the nuances involved in data collection, annotation and further processing. Having said that, in the real world, it also helps to just start collecting data so that some of the unforeseen issues (unknown unknowns) show up as early as possible and iterate over time.
Our data collection effort is spearheaded by a mixture of trained nurses, Auxiliary Nurse and Midwives (ANMs) and Accredited Social Health Activists (ASHAs) spanning hospitals, primary health centres and community visits across ~60 locations pan India. We collect 2D video data using a generic low-cost smartphone accessible to our data collectors and for each baby, record weight, height, chest circumference, head circumference and arm circumference which represent the target variables we wish to predict during evaluation.
The data collected is processed through a data pipeline where anonymization, human in the loop validation, visualization, annotation, and further data processing is done to make the data usable for modelling purposes. This also updates the status of every step to a dashboard in real-time so that members in the team can monitor progress and identify issues if any. This effectively reduces the feedback cycle.
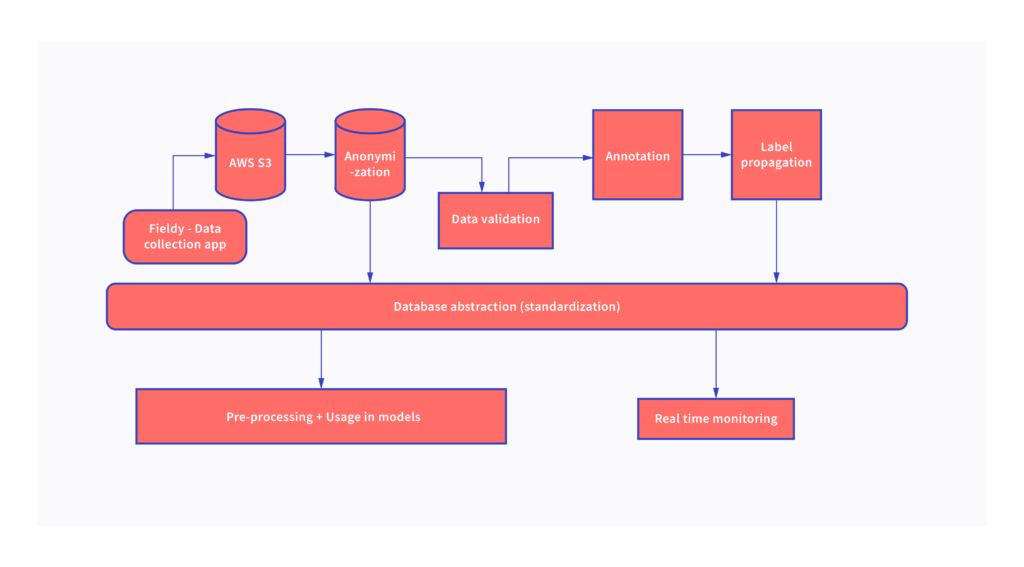
The overall system diagram of our data pipeline is shown above. All our data is collected using a mobile app built in-house dubbed Fieldy using which we collect information about the baby and corresponding 2D videos. The collected data goes through an automated pipeline where sensitive data is anonymized and the videos are converted into sequences of images and copied over to appropriate directories on our servers. We set up a data visualization portal where team members and our manual data validation team can go through the data and annotations systematically and provide feedback that then feeds into the data collectors’ retraining at regular intervals. We check the collected data with a checklist we prepared using the visualization portal. Keypoint annotation is done for frames at a regular interval and interpolated to annotate the entire video using label propagation algorithms. All the information is then standardized at our database abstraction layer so that data we collect from about 60 sites is normalized and is queryable while also allowing us to monitor various blocks in the pipeline individually.
Challenges
For the kind of work we do at Wadhwani AI, more often than not we end up having to collect our datasets from the field which adds another layer of complexity as opposed to crawling the internet to collect data and processing it. Collecting and curating a custom dataset is hard, especially when the effort is distributed i.e. data being collected from multiple locations and states simultaneously by multiple co-ordinators, and when the data collectors have comparatively little digital literacy.
We faced a variety of challenges some of which we couldn’t anticipate at the get-go. We’ve listed down some of the challenges below
- Video quality not matching the desired quality
- Blurry videos
- Cropped videos
- Multiple babies within the same video
- Lighting and shadows
- Annotation quality not matching our desired quality
- Differences in the data collected from various sites and standardization
- Improving the pipeline without affecting data collection
Where we are now
We spent some time at the beginning of data collection in training the data collectors, guiding them on what valid/invalid videos look like and other instructions to ensure that the baby is not cropped out during the filming. We also have a training session when onboarding new data collectors and annotators, where someone from the team typically gives a tutorial on expected criteria and addresses their questions in the process.
The most important piece though, came through when we began scaling our data collection process. We made a design choice to build everything off a centralized database that not only allowed real-time tracking of each step of our data pipeline but also was instrumental in the automation of the data pipeline.
We can track the data collected daily, automatically send unannotated data for manual annotation, do basic sanity checks and track all the information via the master database in real-time to allow members of the team to track progress and make other adjustments if necessary. Recently we built a comprehensive data visualization tool that allows us to view the videos being collected via the browser, allows overlaying the manual and algorithmic annotations so that we get a qualitative view of our data as well as annotations. We can also query the database to find the weight distribution, gender distribution, location distribution of the data as well as inspect the annotations, track the annotators who annotated a particular frame etc., all of which has proven to be extremely beneficial to our Products and Programs team.
Investing time in the data pipeline has proven to be extremely useful in scaling our data collection efforts. We have now collected about 5k videos amounting to roughly 3M images from 4 different states in India. We have annotated over 150k images manually and have used algorithms to propagate annotations across frames within a video.
Our engineering team is using some of these learnings in building a generic data processing and automation pipeline to use across all our projects at WIAI.