The world has changed. And it is not just social distancing and masks. Covid-19 has acted as an accelerant for new tech-enabled solutions entering industry. Agriculture has started to see changes as well. So, we at Wadhwani Institute for Artificial Intelligence look at three innovations that will change agriculture. For the sake of simplicity, we have split each of these into three parts.
- What: The specific change we see happening.
- Why: Why this change needs to happen, the opportunity around it and the reason why it is likely to succeed.
- Challenges: The speed bumps along the way.
E-commerce
What: In an article by our colleague, Vasudha Khare, we detailed how farmer movements were restricted and due to the lockdown so were her options when it came to sellers. A farmer we spoke to sold so his crop wouldn’t rot and all he cared about was making back his cost. Though his problem was exasperated by the virus, it is a challenge that farmers across the world face on a regular basis. An e-commerce platform would mean farmers would be able to get access to customers and transparently set pricing. Farmer collectives would also find a place on these platforms where they could bulk sell their products to industry at good prices.
Why: One of the biggest challenges for farmers during sowing is the pressure of finding a good customer when she is ready to sell. E-commerce could facilitate buyers right from the sowing stage. This would not only help farmers with liquidity but also give them an idea of the input costs that would be needed to make a profit. A supermarket, for example, could be a farmer’s buyer and could establish a supply chain from the farm to its store, which would cut down on costs and increase margins. In the case of organic and niche products such as flowers, it would also give direct access to end-users thus removing middlemen, making things affordable.
Challenges: Placing early orders is a double-edged sword. Farming, at the end of the day, is a speculative business. The farmer relies on several factors beyond her control—weather, pests and animals. All of these factors can affect yield. So if a farmer promises say X kg of oranges at the end of the season and are hit by unseasonal rain, she may not be able to meet the order. Insurance as an answer has been pitched multiple times but the need of the hour is an innovative product, which calculates not just the output of the region but also the farmer, the type of soil and can predict changes in weather systems.
Apart from this, the farmer will either have to tie up with a big e-commerce company to ship her orders or will have to do it herself. Both cost money. Most e-commerce companies charge steep commissions to facilitate sales. Preset prices may artificially control prices, which will cause roadblocks for the farmer.
IoT and Decision making tools
What: Essentially, it is about digitizing farms. Agriculture has so far remained an industry that has not been able to adopt technology and the internet. Here technology will be able to allow farmers to not only make informed decisions such as knowing when a swarm of pests could attack her crop and the preventive measures that can be taken, to release the right amount of pesticide at the right time. Across the world, water is a very precious commodity. In agriculture, more so. Knowing exactly how much water a crop needs and when the best time to water it could be evaluated by AI. A few companies have started to make headway in this sector around the world.
Why: Let’s take spraying pesticides as an example. Doing this alone is not the answer, but also knowing exactly how much to spray and at what time. Not only are pesticides expensive but they can also cause long term damage to soil composition. This means the farmer will have to either add more fertilizer or see yield decline, year on year. Additionally, pesticides are harmful to the health of the farmer and her family. Wadhwani Institute for Artificial Intelligence is working on a tool, which is being tested in collaboration with cotton farmers in Wardha, that could not only predict when a pest attack may occur but can identify pests from traps. This would mean lower costs for the farmer, potentially higher yield and the ability to make better decisions.
Challenges: Availability of the internet. Despite increasing internet coverage in the country, about 550 million people in India use feature phones. This means most phones cannot connect to the internet. Using apps is an uphill battle. Add to that the cost difference between a feature phone and a smartphone. An average feature phone costs $16 (Rs 1,200) whereas a smartphone costs $66 (Rs 5,000). There is still some reluctance in farmers to use technology instead of knowledge passed down over generations. This can be overcome if the government, not-for-profits and industry pitch in to convince farmers to take to technology.
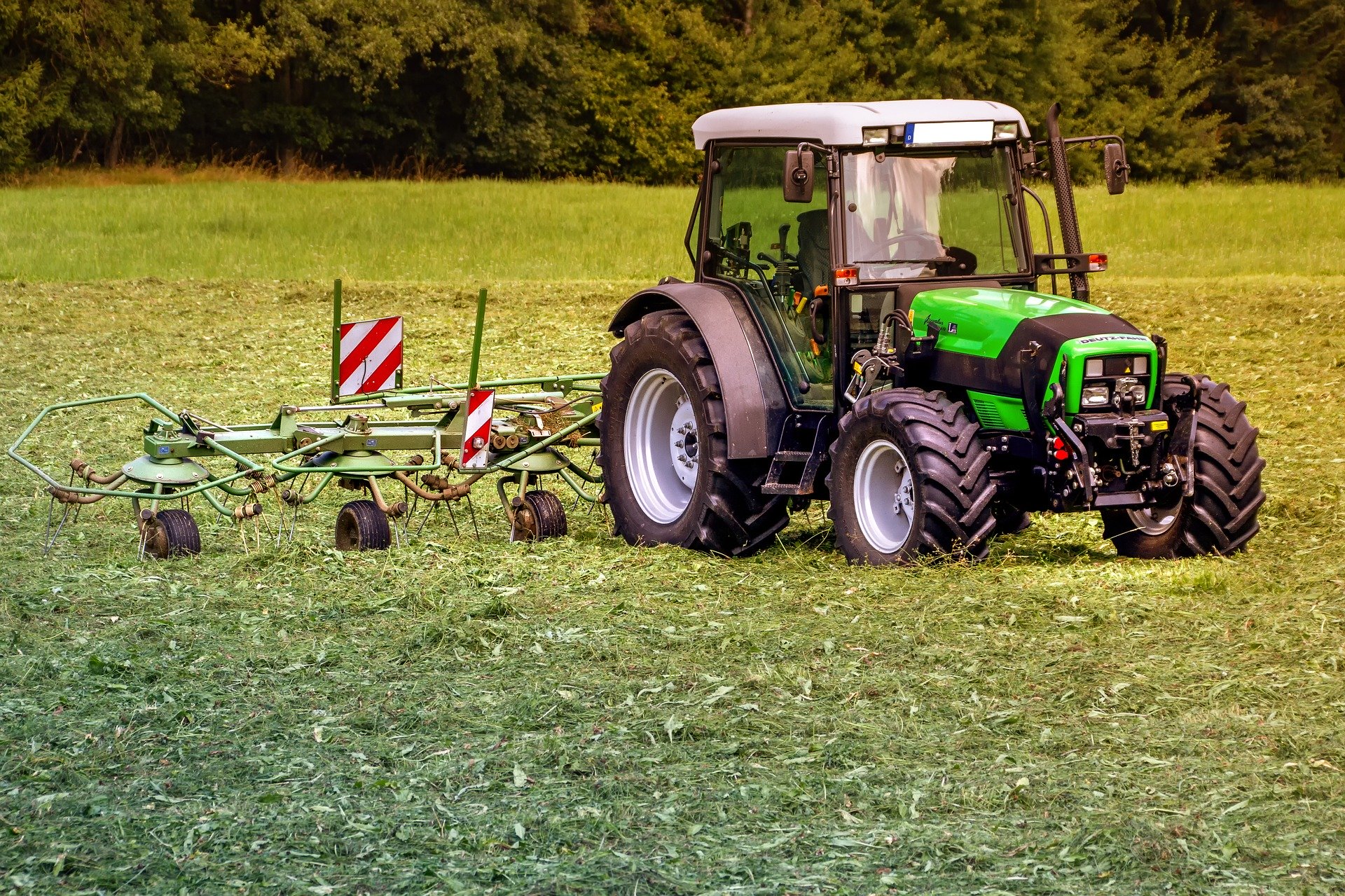
On-demand farm equipment
What: The market for farming equipment in India is set to be valued at almost $12 billion by 2024. But those figures may not be realised, especially with the COVID crisis and locust attacks on farms across the country. This means farmers will struggle to recover input costs. So far, governments, both central and state, have tried to make debt easily available. Farm equipment loans have been difficult to obtain and in the time of a crisis such as this, farmers may opt to defer major capital expenditures. It will mean farm equipment manufacturers may find themselves with unsold stock. However, the popularity of on-demand services such as Uber and Swiggy may hold an answer.
Why: There are two solutions. One, farm-level entrepreneurs with existing equipment could coalesce on a platform and lease out farm equipment when needed. Two, farm equipment manufacturers have a well-established network of showrooms and distribution centres in most districts in India. This will give farmers easy access to machinery that they need for short bursts of time. Think of it as tractor or planter rentals. It could effectively replace manual labour or using livestock. It will be a non-capital intensive expenditure. If used correctly, it could boost yield and give farmers better margins. For industry, this would mean it could open up a new stream of revenue as well as utilise unsold inventory, which usually weighs down the books of manufacturers. After use, this equipment could be available to the farmers at a lower cost. This could make purchases palatable to some customers. A mix of the two, not unlike Ola or Uber’s model in India, could be the way forward.
Challenges: The challenges in this solution are two-fold–operational and business model. Both can be solved. Let’s look at the business model challenges first. Farm equipment is usually sold at thin margins. So, the ability to compete and attract customers may be limited because the price variations will be narrow. This brings with itself some nuances–pricing. These rentals will have to be very well structured. If it is too high, the farmers may not take to it, if the price is too low, cost recovery could be made difficult. Finding the optimal price will require some tinkering. There is also a fear of cartelisation, which can be solved by government oversight. Next is operational challenges. Not all farmers will know how to operate the equipment. Some may need to be trained. This training will mean additional expenses. Apart from training, the risk of damage may have to be borne by the manufacturer. Insurance products won’t cover this. Repeated and extensive training programmes by industry, not-for-profit and government may help minimize these damages. Manufacturers could also use tertiary decision-making tools such as the size of the farm and age of the farmer to come up with solutions. AI could be a method to find solutions.