The annual TB report declared that the disease claimed over 73,000 lives in 2019 alone. In an earlier post, we explained how India handles TB. A few weeks ago, my colleague spoke about how the country needs to focus on Line Probe Assay (LPA) and technology that can bring efficiency to a mostly manual process. In order to achieve the target to eliminate TB by 2025, set by the National TB Elimination Program (NTEP), we need to rapidly scale the capacity of universal drug sensitivity testing. Our project aims to help scale universal drug sensitivity testing by automating interpretation and tabulation of LPA results.
Today, let’s talk about the solution we are developing at Wadhwani Institute for Artificial Intelligence.
But first, let me tell you about one number.
It can take up to an hour, in some cases, for a lab technician to read, interpret and tabulate a sheet (usually containing 24 strips) of LPA test strips. The lab technician looks at each strip, called LPA. The interpretation helps her identify effective medications for a particular strain of TB bacilli. With labs having around two to three sheets everyday, this translates to two to three humanhours that could have been better spent somewhere else.
The image above shows a typical sheet containing 24 test strips. A lab technician examines each strip and interprets results based on the activated bands. Each interpretation is part of four lakh diagnoses made every year. A number that is expected to double in 2022.
The pluses and minuses we see on the right-hand side of the sheet are part of the lab technician’s interpretation. However, there can be human errors in interpretations. Human errors are not out of the ordinary, but AI can help eliminate these errors.
Let’s look at the image again. Each row in the image’s centre is a test strip that the AI agent needs to interpret. The vertical lines on the strips denote a particular band being present. Based on the activated bands, pluses and minuses are assigned, dictating what medications would work and what will not.
Creating a solution to solve this challenge requires us to know if AI can read and interpret strips. We conducted a simple project on trying to automate this part of the process. It turns out that AI can read them and help us.
AI’s Role
Interpretation requires solving a three-stage problem; locating test strips, reading activated bands on test strips, and mapping the TB variant.
Stage 1: Locating Test Strips
We observe that strips are very structured objects because of bands. We also notice strips are pasted in equidistant assigned boxes. Using these bits of information, we first find spaces (printed boxes) where strips can be pasted and then use template matching to ascertain if a strip is present at that location. We locate the printed boxes by dividing the space between the two anchors (as shown below) into 24 equal parts. We then use multiple cut-outs of strips as templates in template matching.
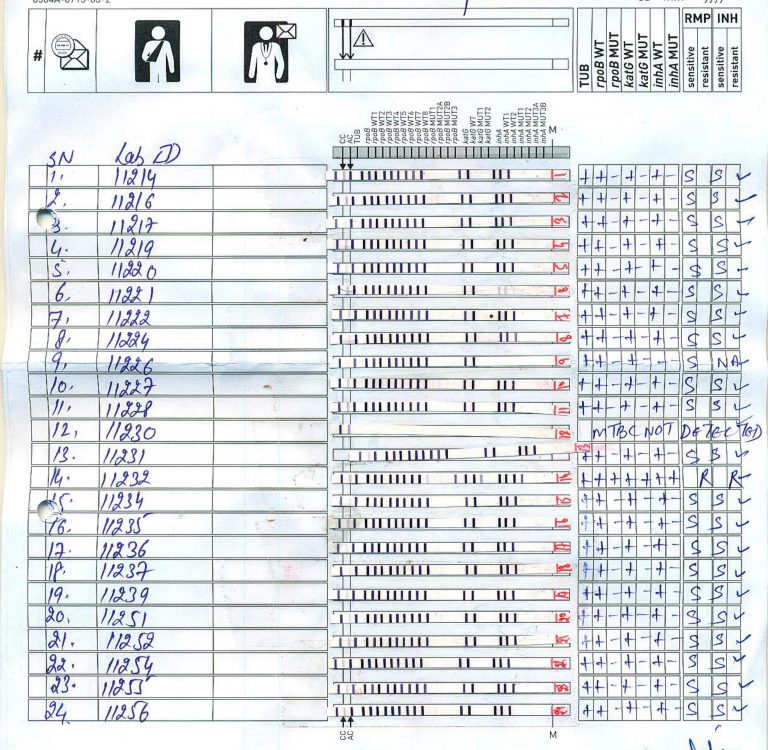
Stage 2: Reading Activated Bands
Once we have located the strips, we use edge detection algorithms to perceive edges. These edges ideally correspond to the activated bands on the test strip.
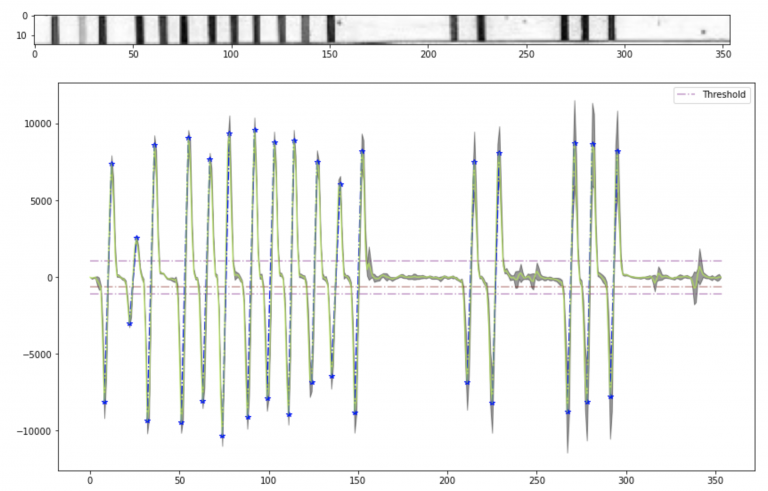
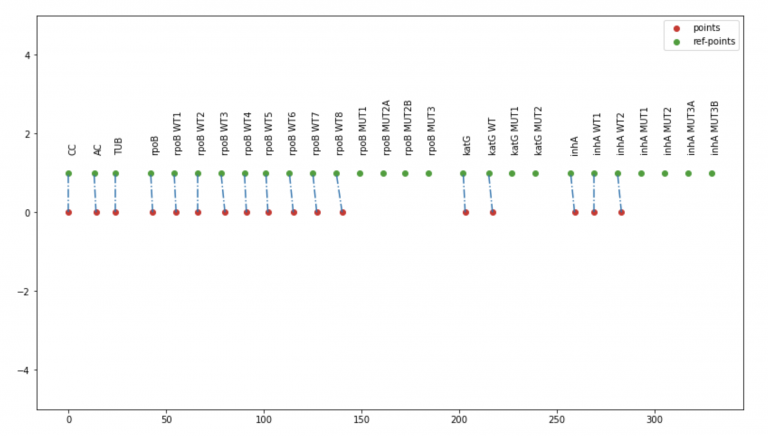
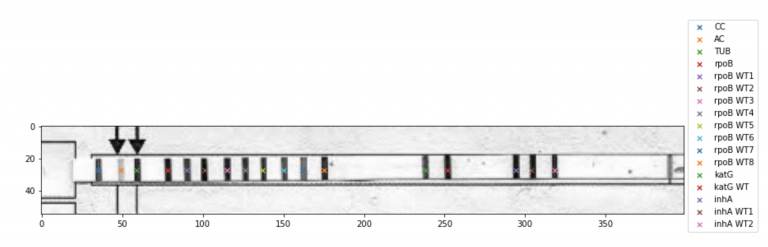
Stage 3: Mapping TB Variant
The last step is relatively straightforward. To call out the TB variant based on the activated bands, lab technicians use deterministic decision rules once they are sure of the activated bands. These rules help them either call out the TB variant or detect any preparation problem in the test.
Our project’s scope was to verify if an AI agent can interpret and help lab technicians save time. Initial results on the test set tell us that this is possible.
But taking this AI piece and deploying it is another enormous challenge. Which is why we are building a human-in-the-loop solution. To do so, we need to think about the tool’s design, the tool’s role in existing workflows, and building trust between the user and the tool through explainability/uncertainty measures. Other more programmatic considerations also need to be fleshed out like the accountability of the decisions made in presence of the tool, the infrastructure capability and the technologies available on ground. But that is for another day.
This solution is being developed under the TRACE-TB project, funded by USAID.